Project
As humans, we naturally adapt our decisions based on how confident we are in our own perception and understanding. Analogously, trustworthy computer vision systems should express an appropriate level of uncertainty when making predictions, such that we can identify and understand their mistakes.
A large portion of research in computer vision is focused on pushing the boundaries of model performance, without addressing the question “are you sure?”. While deep neural networks (DNNs) continue to show unprecedented results across many image understanding problems, they cannot reliably express their uncertainty when faced with unfamiliar or ambiguous inputs.
The goal of this project is to develop methods for capturing uncertainty in DNNs to enable reliable failure detection or out-of-distribution detection for computer vision. The project also aims to explore how uncertainty can be used to improve a model’s predictions.
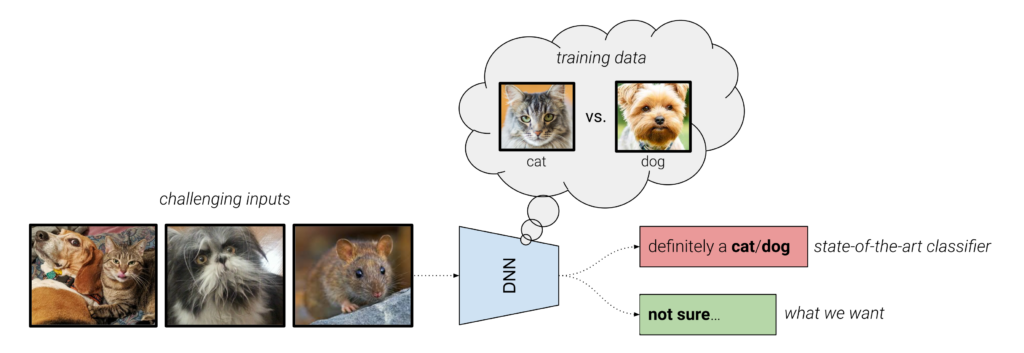
Scientific Work
Beyond AUROC & co. for evaluating out-of-distribution detection performance
Humblot-Renaux, G., Guerrero, S. E. & Moeslund, T. B., aug. 2023, 2023 IEEE/CVF Conference on Computer Vision and Pattern Recognition Workshops (CVPRW).IEEE, s. 3881-3890 10 s. (IEEE Conference on Computer Vision and Pattern Recognition Workshops (CVPRW)).
Funding
This work is supported by the Danish Data Science Academy, which is funded by the Novo Nordisk Foundation (NNF21SA0069429) and VILLUM FONDEN (40516).
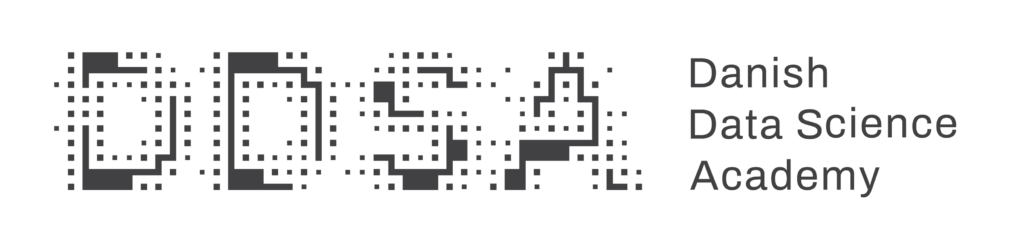
Contact
PhD Fellow: Galadrielle Humblot-Renaux
Email: gegeh@create.aau.dk
Supervisor: Thomas B. Moeslund
Email: tbm@create.aau.dk
Co-supervisor: Sergio Escalera
Email: sergio.escalera.guerrero@gmail.com