Project
The supply sector is subject to ever increasing demands for service and optimization. The large amount of data collected in the sector entails a great opportunity for utilization of machine learning and data science in order to meet these demands. This industrial PhD project aims at investigating how machine learning can create value in the water sector. Furthermore, the goal of the project is to perform research within specific use cases.
Scientific Work
Data-Driven Drift Detection in Real Process Tanks: Bridging the Gap between Academia and Practice
Hansen, B. D., Hansen, T. B., Moeslund, T. B. & Jensen, D. G., 16 mar. 2022, I: Water. 14, 926, 20 s.
Comprehensive Feature Analysis for Sewer Deterioration Modeling
Hansen, B. D., Rasmussen, S. H., Uggerby, M., Moeslund, T. B. & Jensen, D. G., 17 mar. 2021, I: Water. 13, 6, 19 s., 819.
Prediction of the Methane Production in Biogas Plants Using a Combined Gompertz and Machine Learning Model
Hansen, B. D., Tamouk, J., Tidmarsh, C. A., Johansen, R., Moeslund, T. B. & Jensen, D. G., 1 okt. 2020, Computational Science and Its Applications – ICCSA 2020 – 20th International Conference, Proceedings. Gervasi, O., Murgante, B., Misra, S., Garau, C., Blecic, I., Taniar, D., Apduhan, B. O., Rocha, A. M. A. C., Tarantino, E., Torre, C. M. & Karaca, Y. (red.). Springer, Bind 12249. s. 734-745 12 s.
Sewer Deterioration Modeling: The Effect of Training a Random Forest Model on Logically Selected Data-groups
Hansen, B. D., Rasmussen, S. H., Moeslund, T. B., Uggerby, M. & Jensen, D. G., 2020, Knowledge-Based and Intelligent Information & Engineering Systems: Proceedings of the 24th International Conference KES2020.Elsevier, Bind 176. s. 291-299 9 s.
General Sewer Deterioration Model Using Random Forest
Hansen, B. D., Jensen, D. G., Rasmussen, S. H., Tamouk, J., Uggerby, M. & Moeslund, T. B., 2019, IEEE Symposium on Computational Intelligence for Engineering Solutions (IEEE CIES).IEEE, 8 s.
Funding
The project is funded by EnviDan A/S and Innovation Fund Denmark.
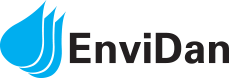

Contact
PhD-Student: Bolette Dybkjær Hansen
Email: bdha@create.aau.dk
Supervisor: Thomas B. Moeslund
Email: tbm@create.aau.dk